We all know about terms like machine learning, natural language processing, computer vision, and neural networks that allow artificial intelligence models to function properly. Similar to these techniques is Deep Learning, which is used in most of the AI models that industries and commoners use in their daily lives.
In this blog, we will read about deep learning, what it is, why it is, and its applications and benefits.
Table of Content
What is Deep Learning?
Deep learning is a form of neural network and machine learning that allows computers to function like the human brain. It helps the machines perform complex tasks like driving, virtual reality, and speech recognition image processing and supports the functioning of NLP and CV models.
How Deep Learning Works?
Deep learning models or neural networks are programmed like the human brain. Similar to neurons in the human brain that interact with each other to pass information signals, NN models have artificial neurons or nodes that are present in layers to process the user input.
The neural network model has 3 layers: Input, Hidden, and Output.
Input layer takes the user input and passes it forward to get processed.
Hidden layers process the information and forward the final result to the output layer. Here, most of the processing happens. Hidden layers are 1 or more in different neural networks.
When the number of hidden layers is two or more, it becomes a deep learning model.
Output layer receives the processed result from hidden layers and presents the final output to the user.
All the network nodes have specific weights and thresholds. The weights determine the importance of input signals, and the thresholds determine whether or not the node is activated. The node becomes active when the combined signals to the node exceed its threshold. The node gets activated and passes the information to the next layer.
Deep Learning Applications
The following are the deep learning applications that depict how deep learning is embedded in people’s everyday lives.
Scam Detection
Deep learning models are well-trained to identify patterns in the datasets. Using this learning, they recognize the patterns of scams and fraudulent activities and thus detect them as soon as possible. Moreover, any suspicious login attempts or identifying weak passwords that might be vulnerable to hacking are alerted to the user to prevent unusual activities.
Customer Service
All the virtual assistants and chatbots that handle customer queries have deep learning algorithms integrated into their systems. These algorithms allow the bots to understand what the user is saying and act on it. They can forward the query to the respective customer handling team if it is complex.
Finance Services
Whether analyzing trends, forecasting, giving insights on investment strategies, or keeping records, deep learning in finance is a great tool to streamline operations and improve the accuracy of tasks. Moreover, predictive analytics helps study stock trading trends, and loan approvals by considering the past profile of applicants.
Natural Language Processing
Deep learning algorithms also supports the working of natural language processing (NLP) models., and enable them to interpret text and voice inputs. NLP and DL combine to let the bot understand the context of words in the input. Today, chatbots are the most common AI example used in many industries.
Facial Recognition & Image Processing
Being a master of doing complex tasks, DL models can recognize different faces even when the person is in motion or has various expressions. This feature is important in multiple industries like crime investigation departments, surveillance, and HRM in companies. Apart from this, DL models are embedded in most of the image processing, and computer vision (CV) models, to let computers identify and recognize the difference among various visual inputs.
Self-Driving Cars
Self-driving cars are the perfect example of defining the level of complexity the deep learning model can handle. From operating the vehicle to tackling different situations on the road, DL enables the autonomous car to detect traffic lights, recognize signs, and care for pedestrians.
Predictive Analysis
Deep learning models can analyze large datasets and study the underlying trends and patterns. Using these analytics, they can produce future trends and predictive insights, which help business managers make various informed decisions like product development, manufacturing, sales strategies, inventory management, product development, and more.
Recommendation System
Recommendation systems are quite common these days and are used by various e-commerce websites, video streamlining platforms, and music systems. Using deep learning techniques, recommendation systems study customer’s behavior, monitor their daily habits, and then recommend the products, services, music, movies, and series that best suit the user’s interests.
Healthcare
Deep learning in healthcare assists doctors in streamlining medical image analysis and helps in screening more patients in less time. However, DL is embedded in AI-backed hospital management software that automates the data entry and patient record management work, reducing pressure on hospital faculty.
Automation
Deep learning backs the ability of AI to automate manual and repetitive operations to streamline operations of different types of industries. Thus, automation is one of the reasons for the wide adoption of artificial intelligence (AI).
Benefits of Deep Learning
The following are the benefits of deep learning:
Automatic Feature Learning
Deep learning models can automatically identify and extract important features from raw data without manual feature engineering. This saves time and effort while enhancing model performance.
Pattern Discovery
DL models can analyze large data sets and uncover hidden patterns. Using that information, a DL model can even produce insights for which they have not been trained.
Handling Complex Data Sets
In today’s digital age, data is being produced every day in various forms and with increasing levels of complexity. DL algorithms can smoothly handle all the large, complex, and varying datasets.
Versatility with Various Data Types
Deep learning is adaptable to different types of data, including text, images, audio, and time-series data, making it useful across a wide range of applications.
High Accuracy
Deep learning models often achieve higher accuracy than traditional machine learning models, particularly in tasks involving large amounts of data and complex patterns.
More Effective Than Other ML Models
The reason why DL models are preferred for most of the operations is that DL models are more accurate and outperform other ML models in terms of efficiency, complexity, and scalability.
Hence, deep learning algorithms play a huge role in wide adoption, and popularity of artificial intelligence (AI). The ability of deep learning to mimic human brain’s neural networks enables machines to perform complex and complicated tasks which basic AI models might fail. As AI paves the way to more advancement, we can expect some more enhanced and flexible versions of deep learning that can introduce some amazing AI deals to us in future. Till then, let us enjoy what we have now, and make the most of it!
If you are looking to develop any DL-backed AI solutions, go no where now as Build Future AI, has got it all covered. Having successfully delivered many deep learning and AI projects, we have extensive in this field.
Reach out to us via email: business@buildfuture.ai and grow your business with AI!
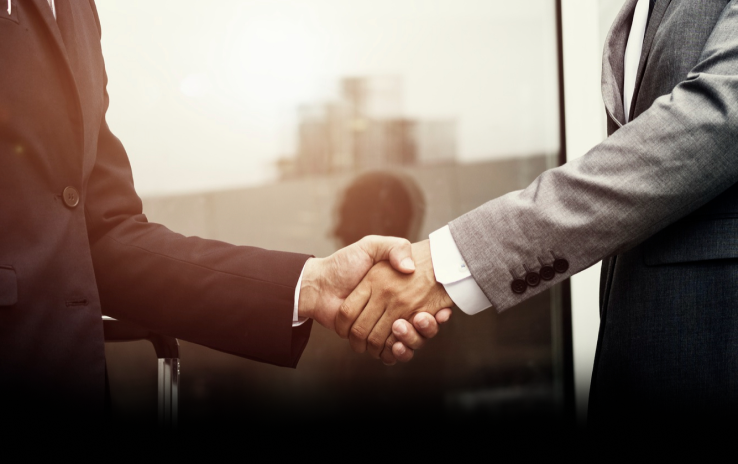