When we think of learning something, it basically involves grasping the concepts and retaining them in our minds, but what about forgetting? Does forgetting things help in learning in any way?
Consider your own brain for a moment, over the time, it filters out unnecessary information and only remembers the valuable ones. Just as humans selectively remember and forget, artificial models can also do the same to enhance their learning ability.
In this blog, you’ll delve deep into a game-changing method of forgetting called “Selective Forgetting,” used to enhance the learning capabilities of AI models.
What is Selective Forgetting?
Selective forgetting refers to the periodic erasing of information to allow AI models to learn new languages easily and quickly. This approach is similar to how our brains work. Let’s discuss how.
About Artificial Neural Networks
Most of the AI language models today are based on artificial neural networks. Neural networks are machine learning algorithms that work similarly to the human brain. Neurons, the fundamental units of the human brain, receive and pass signals from one neuron to another. Likewise, artificial neural networks have artificial neurons that have specific threshold values and weights. When an artificial neuron reaches the threshold, it passes the information to the next neuron. Initially, the flow of information may be random, but this improves as the neural network adapts to the training data set. For an example, if an AI researcher wants to create a bilingual AI model, it will feed the training data having text in both languages into the language model. Eventually, the model will adapt and adjust the connections between neurons so that words having the same meaning in both languages would get related to each other.
However, training any AI model requires a lot of computing power. But what’s even harder is its retraining. Suppose you trained a language model on 100 languages and now want to add a new one; you’d have to retrain it entirely, which is quite expensive and time-consuming. This is when Selective Forgetting comes into play.
How Does Selective Forgetting Work?
A neural network is made of layers, and in the language model, the building blocks of the language are stored in the first layer, called the embedding layer.
To learn a new language, the information stored in the embedding layer of the AI-model is erased, and it is re-trained with the tokens of a new language. The underlying concept is that while the embedding layer stores the information of words used in a language, the deeper layers store more abstract information about the ideas behind the human language that help the model learn a new language easily. Humans conceptualize the same things in different words. For instance, an apple is just a word for something sweet and juicy. Hence, it may be called something else in another language.
Even though the process of training, erasing, and re-training seems simpler, it still requires a lot of language data and computing power. Thus, the idea of periodic erasing of the embedding layer arose, and using it, the machine became used to resetting, and the process of adding any new language became easier and faster.
Case Study of a Language Model- Roberta
A common language model- Roberta was retrained using both the ways, selective forgetting approach, and the standard, non-forgetting approach. The results were compared of the two cases:
Initially, the language accuracy score of the model was measured when it was trained for one language with a full training data set of 70 billion tokens using both the periodic forgetting technique and the standard technique. The selective forgetting technique performed a little worse than the conventional one, receiving a score of 85.1 as compared to 86.1 in the other.
The models were then retrained with new language using a much smaller dataset of 5 million tokens. Now, the score dropped to 62.7 for the selective-forgetting method and to 53.3 for the standard method. Hence, in this case, the selective forgetting model performed much better.
Later, when the computational limits were imposed, and the training length was cut from 1,25,000 steps to 5000, the accuracy of the forgetting model decreased to an average of 57.8. On the other hand, the standard model plunged to 37.2. Below given chart shows the stats:
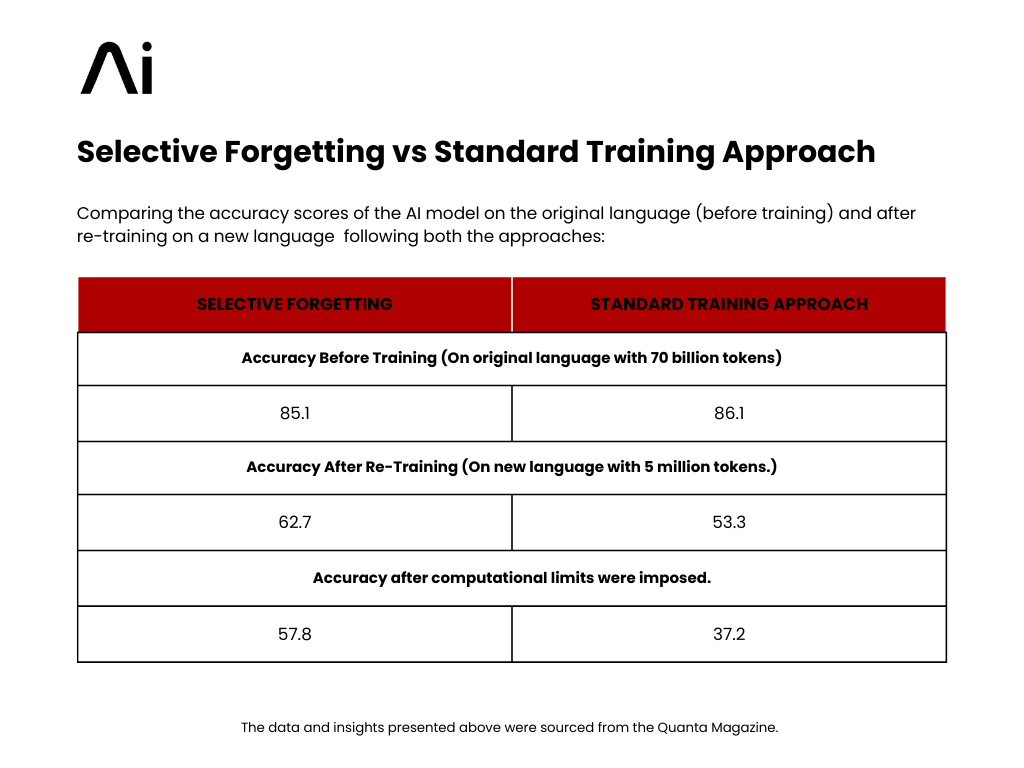
Thus, the stats clearly show how impactful the selective forgetting technique is in modifying the learned concepts in AI models.
The data and insights presented above were sourced from the Quanta Magazine.
Conclusion
When it comes to the concept of artificial intelligence it refers to incorporating human-like capabilities into machines. However, selective forgetting is another side that takes this incorporation to deeper levels. This technique relates to putting the adaptive capabilities and flexibility of the human brain into machine algorithms in order to enhance its learning ability and make it more flexible to learn new concepts with time. As the world of AI develops, we can write on a rock that this method of periodic forgetting or resetting will open new doors, clearing the path for new insights and adaptations.
Looking for the best AI development, integration, and consulting company? Look no further because Build Future AI has it all!