What Is AI in Genomics?
AI in genomics refers to the application of artificial intelligence – particularly machine learning models and sometimes more specialized models like deep learning – to the vast sets of data generated when sequencing and analyzing genomes. In simple terms, think of it like having a super-powered microscope for digital data: it sifts through huge quantities of genetic information, spots meaningful features, and even predicts outcomes, all in ways that human researchers alone would struggle to match. Genomics itself involves the study of an organism’s entire set of DNA, including genes and their functions. So when you tie AI to genomics, you’re essentially turbocharging the rate at which researchers can understand genetic variants, link those variants to diseases, and design medical interventions. A big part of why this matters is that genomic research these days is generating massive amounts of raw data. Imagine tens of millions of lines of raw code representing every letter of DNA in your body, plus another set for your neighbor, plus entire sets for thousands (or hundreds of thousands) of other participants. Storing that data is challenging enough, let alone analyzing it. That’s where AI comes in. Speaking with knowledgeable advisors could be a good idea if you’re ever unsure about how AI might integrate into your clinical research or biotech project. In addition to saving months of trial-and-error, working with a team that is knowledgeable with AI in genomics can reveal new applications for genomics data that you may not have thought of.Accelerating DNA Sequencing Through AI
DNA sequencing has come a long way since the days when mapping even just one human genome took years of painstaking work. Today, AI-driven DNA sequencing tools help transform a complex, time-consuming process into something more rapid and accurate. Researchers feed raw sequencing data into machine learning pipelines that automatically “clean” noisy data, piece together fragmented reads, and spot anomalies. Let’s be clear: we’re not at some magical point where everything is instantaneous, but the improvements in speed and cost are significant compared to older methods. Here’s how it often goes: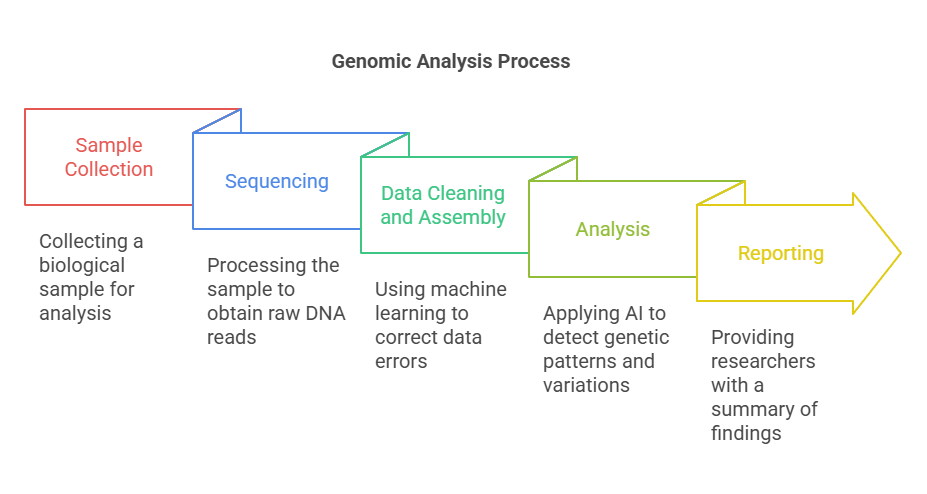
- Sample Collection: You start with a biological sample (let’s say a blood sample).
- Sequencing: The sample is processed using modern sequencing hardware that outputs raw reads of DNA.
- Data Cleaning and Assembly: Machine learning in genomics tools step in to correct errors in the raw data. (This used to require painstaking manual oversight.)
- Analysis: AI algorithms detect patterns, identify variations, and map those variations to known phenotypes.
- Reporting: Researchers receive an analytic summary that highlights crucial genetic markers relevant to diseases or traits of interest.
Aspect | Traditional Workflow | AI-Driven Workflow |
---|---|---|
Data Processing Speed | Moderate (heavy manual oversight) | Faster (automated cleaning and error-checking) |
Error Detection | Mostly manual or semi-automated | Largely automated with machine learning |
Entry Skill Level | Requires domain experts at every stage | Allows integration of broader team skill sets |
Insight Generation | Slower, dependent on experts’ knowledge | Quickly surfaces complex patterns in data |
Overall Cost | Higher due to longer timelines | Decreasing due to automation and faster results |
Machine Learning in Genomics for Disease Prediction
Think about all the health mysteries that remain unsolved. Many revolve around understanding which genetic changes lead to diseases like cancer, heart conditions, or rare genetic disorders. Traditionally, finding these disease-linked genetic variants involved large-scale studies and significant guesswork. But with machine learning in genomics, you can train predictive models to spot disease markers far more precisely. These models don’t just rely on superficial data either. They dig into both coding regions (the sections of DNA that actually produce proteins) and non-coding regions, which historically got less attention. It turns out these “junk” regions can carry meaningful regulatory information that influences gene expression. Here’s the kicker: you don’t even need to predetermine which features of the genome might be most important. Advanced models, particularly deep learning architectures, can learn which features are valuable on their own. They do this by combing through entire genomic datasets and analyzing patterns global in scope and too subtle for a human researcher to notice.Actionable Insights for Clinicians
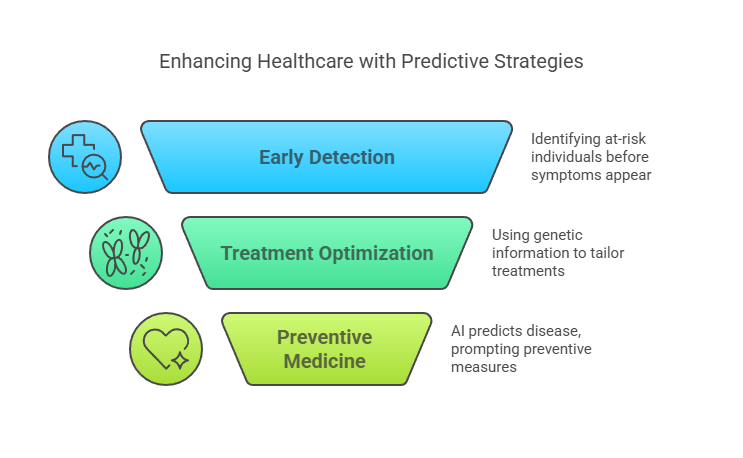
- Early Detection: Physicians can suggest lifestyle modifications or early therapies by identifying at-risk individuals before symptoms appear.
- Treatment Optimization: Genetic information is used to determine which treatments are most appropriate for a certain genetic profile.
- Driving Preventive Medicine:AI-powered disease prediction in healthcare systems can highlight warning signs in patient records, directing care teams to take precautions or repeat tests.
The Rise of AI for Personalized Medicine
The foundation of precision medicine, also known as personalized medicine, is the knowledge that no two people are precisely the same, even if they have the same diagnosis. Although this idea has been around for a while, in recent years, AI for personalized medicine has really taken off. Let’s be honest: the term personalized medicine might sound like fancy marketing jargon. But I can tell you from direct exposure to real-world applications that it genuinely saves time and often leads to better outcomes. AI is now used in precision medicine, for instance, in some cancer treatments. Clinicians can reduce the amount of trial-and-error by determining which drug combinations might be most effective for a patient by examining the distinct genetic signature of a tumor.Personalized Treatment Planning
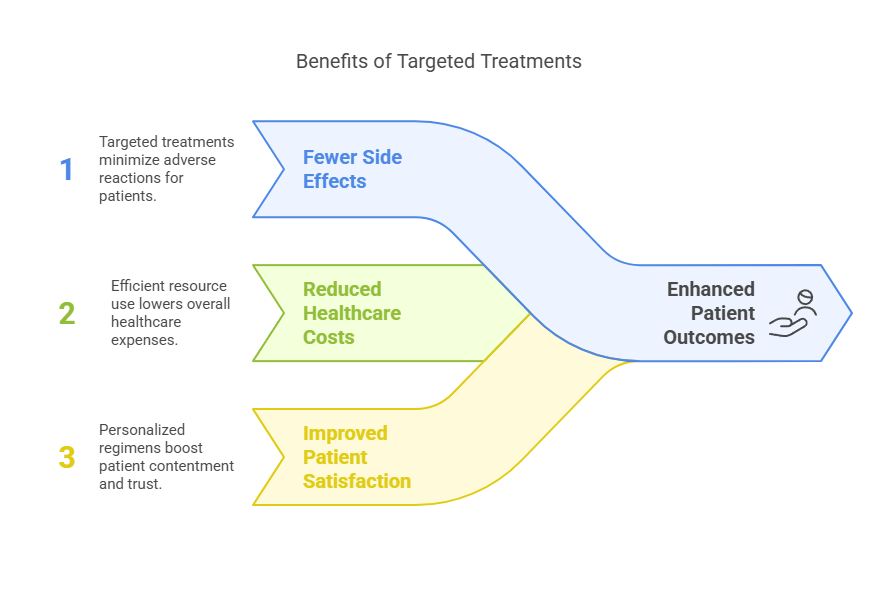
- Fewer Side Effects: Because targeted treatments tend to rely on highly specific mechanisms of action.
- Reduced Healthcare Costs: By focusing resources on the most effective therapy early on.
- Improved Patient Satisfaction: Patients appreciate regimens that consider their unique biology.
AI-Powered Gene Editing: Shaping the Future With CRISPR
Gene editing is no longer the domain of science fiction. Tools like CRISPR (Clustered Regularly Interspaced Short Palindromic Repeats) allow scientists to precisely cut and edit DNA at predetermined locations. But how does AI for gene editing come into play? AI helps with gene editing by determining which specific sections of DNA to target. Traditional gene editing experiments typically require laborious trial and error to confirm sequence changes and rule out unintended modifications. But an AI-driven system can scan genetic data, predict ideal editing sites, and model potential (and sometimes hidden) side effects of a given edit. Yes, it can still be tricky, and we’re not at a point where you can casually let an AI pick all your CRISPR targets without oversight. But the combination of AI in biotechnology with CRISPR soared in popularity because it drastically cuts down the time it takes to identify, validate, and test a desired genetic modification. This holds true for both businesses creating novel treatments for inherited illnesses and research labs investigating fundamental genetic processes. It makes sense to think about working with knowledgeable partners if you want to use AI for gene editing techniques in your lab or product pipeline. You may avoid potential problems by taking that step, which includes making sure you have the proper software architecture to handle complex AI workloads and maintaining regulatory compliance.Applications in Biotechnology and Ethical Considerations
AI in biotechnology goes well beyond just analyzing DNA. It also shapes research on protein folding, metabolic pathways, and complex interactions in cellular environments. For startups and established biotech firms alike, the potential to develop new drugs, vaccines, and diagnostics with the help of AI in genetic research is huge. But let’s pause for a second here and talk ethics. When working with genetic data, you’re working with extremely private information that others can’t alter like they could a password. Your whole lineage and health status can be inferred from your DNA, unlike a fingerprint. The risk of misuse is non-trivial. Privacy concerns loom large, and so do questions about consent, data security, and liability when predictions turn out to be wrong. Researchers also confront potential biases in AI models that stem from underrepresented genomic data. For instance, if certain ethnic groups are poorly represented in genomic databases, machine learning in genomics might be less accurate for those populations. This can lead to disparities in healthcare outcomes if left unaddressed. The catch? This is exactly why having robust data governance and compliance strategies is critical. In my years of working with biotech companies, I’ve found that making sure data is handled ethically not only shields your company from legal issues but also builds confidence with partners, community stakeholders, and participants. It might be time to get professional advice if you’re having trouble handling these privacy and ethical issues. Collaborating with Buildfuture can help you chart a responsible path forward, backed by proven frameworks for managing sensitive genomic data.Practical Steps for Leveraging AI in Genetic Research
At this point, you might be thinking, “Sure, this sounds exciting. But what does an actual AI-in-genomics project look like day-to-day?” Let’s outline some of the high-level steps that many biotech teams take to incorporate AI into genetic research: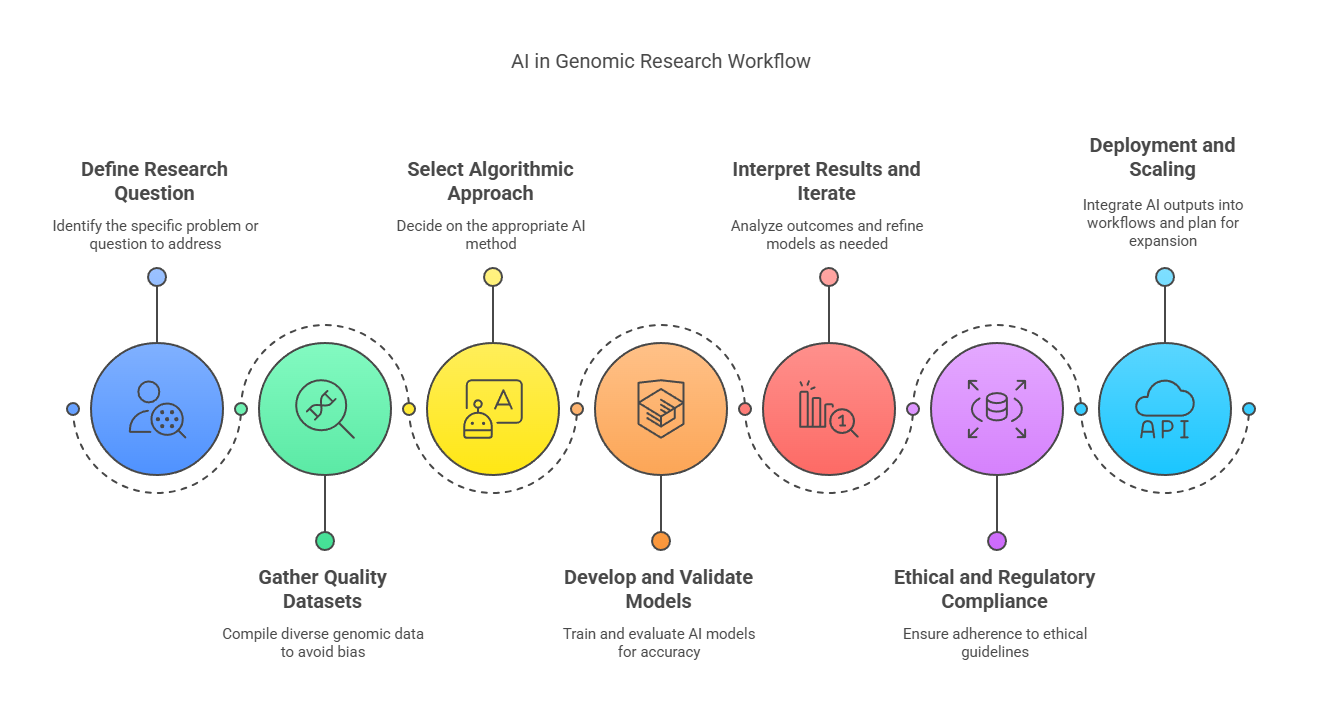
- Define the Research Question Identify the specific problem or question you want the AI to address. Maybe you’re studying a rare genetic disease or looking for biomarkers of responsiveness to a new drug.
- Gather Quality Datasets Compile genomic data from a diverse range of populations if possible. This helps ensure your AI models won’t be biased.
- Select an Algorithmic Approach Decide whether you need simple machine learning classifiers, deep learning for large-scale pattern recognition, or a more specialized approach tailored to your research question.
- Develop and Validate Models Train models on your dataset, then evaluate their accuracy, precision, and recall. Adjust parameters or integrate new data based on these results.
- Interpret Results and Iterate Model interpretation is crucial. Avoid deploying black box models in critical healthcare settings without thorough validation. If an approach doesn’t yield the desired outcomes, refine and iterate.
- Ethical and Regulatory Compliance Ensure data usage follows appropriate guidelines. Obtain informed consent and maintain data anonymity in line with local and international regulations.
- Deployment and Scaling Integrate your AI-driven outputs into research workflows or clinical decision support tools. Plan for scalability if you intend to expand usage across multiple sites.
Advancing Disease Prediction With Big Data Analytics
Integration is a big theme here. AI in genomics doesn’t operate in a vacuum. It often intersects with big data tools that handle everything from data storage and retrieval to advanced analytics. In some cases, organizations adopt cloud platforms that let them pipe in data from multiple clinical trials or research studies. They then run machine learning models to uncover patterns at a much larger scale. One key advantage of merging AI with big data is you can analyze not just genomic data, but also electronic health records, patients’ imaging results, and even social determinants of health. This holistic approach could refine the accuracy of AI for disease prediction and might speed up the route to personalized medicine. Hospitals and pharmaceutical companies are now beginning to realize the potential synergy here. If it all sounds a bit overwhelming, it’s because it can be. Storing history’s largest repository of DNA sequences, then spinning up multiple ML pipelines to cross-reference that data with other sources requires both technical chops and strategic planning. The good news is that Buildfuture has seen this complexity before. We can guide you in building a flexible architecture that scales, whether you’re a small lab or a larger institution.Industry Challenges and How to Overcome Them
No cutting-edge technology is without challenges. When it comes to AI for personalized medicine, AI-driven DNA sequencing, and AI for gene editing, a few hurdles stand out: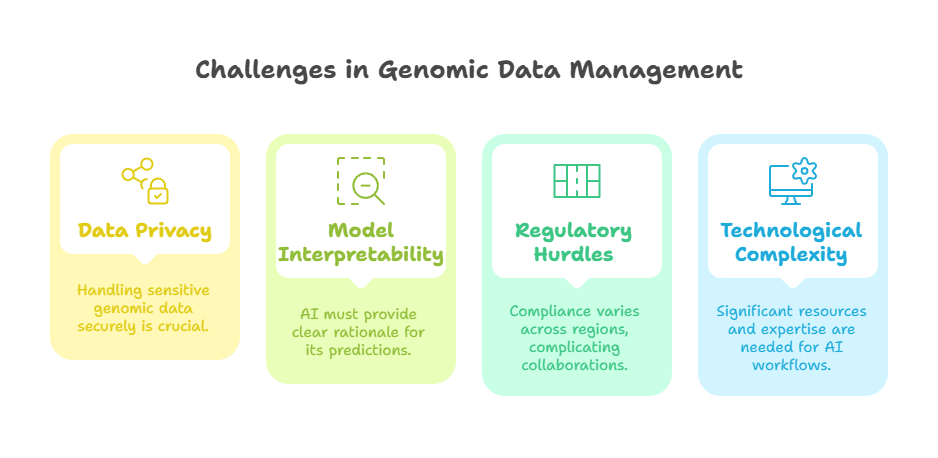
- Data Privacy and Confidentiality: Handling personal genomic data is inherently sensitive. Robust encryption and secure data-sharing protocols are must-haves.
- Interpretability of Models: Clinicians often demand transparency. It’s not enough for an AI to say a patient has a high risk of developing a specific disease – it should also provide some rationale.
- Regulatory Hurdles: Different regions have varying rules on genetic testing and data usage. International collaborations can run into compliance snags.
- Technological Complexity: Running AI workflows at scale requires significant computational resources and specialized knowledge.
Looking Ahead: The Future of AI in Genomics
AI in genomics is still in its early stages, which is part of what makes it so exciting (and occasionally nerve-wracking). As these technologies evolve, it’s likely we’ll see: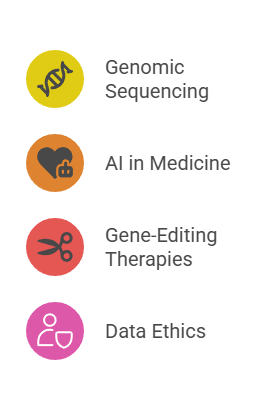
- Faster and Cheaper Genomic Sequencing: Ongoing hardware advancements will make full-genome sequencing a routine part of preventive healthcare.
- Wider Adoption of AI in Precision Medicine: More hospitals and clinics will integrate AI-based tools into their standard practice for a variety of conditions.
- Emergence of Gene-Editing Therapies: With outcomes proven safe and effective, we may see more gene-editing treatments approved for mainstream use.
- Growing Importance of Data Ethics: As data collection scales up, so will public and regulatory scrutiny about privacy and fairness.
Conclusion
At its core, AI in genomics marks a profound shift in the way we approach and understand genetic research. Whether you’re a research scientist seeking new avenues, a hospital aiming to refine predictive diagnostics, or a biotech venture pushing the boundaries of gene editing, the capacity to harness AI-driven insights is a game-changer. Machine learning in genomics unlocks the ability to analyze enormous datasets with unprecedented speed. AI for personalized medicine goes a step further, tailoring treatments to each patient’s genetic signature. And AI for gene editing, with CRISPR at the forefront, is allowing us to design interventions that could one day eradicate inherited conditions. Of course, none of this is straightforward. There are real ethical considerations around privacy, data bias, and equitable access to these innovations. Yet with thoughtful leadership and collaboration, the benefits can outweigh the risks. If you’re ready to leverage AI-powered genetic analysis or AI-driven DNA sequencing, consider forming new partnerships or expanding your existing ones. The door is wide open for visionary efforts that can shape the future of healthcare and biotechnology in remarkable ways. You might want to take the next step by bringing your team together with experts in AI for disease prediction and genetic research. At Buildfuture, we don’t just build products – we form partnerships to ensure you get the guidance, strategy, and technical support you need for long-term success. Feel free to connect with us if you need a sounding board or hands-on help. We’d be happy to discuss how AI in genomics can drive your next set of breakthroughs. It’s time to shape what’s possible for tomorrow’s research and patients today.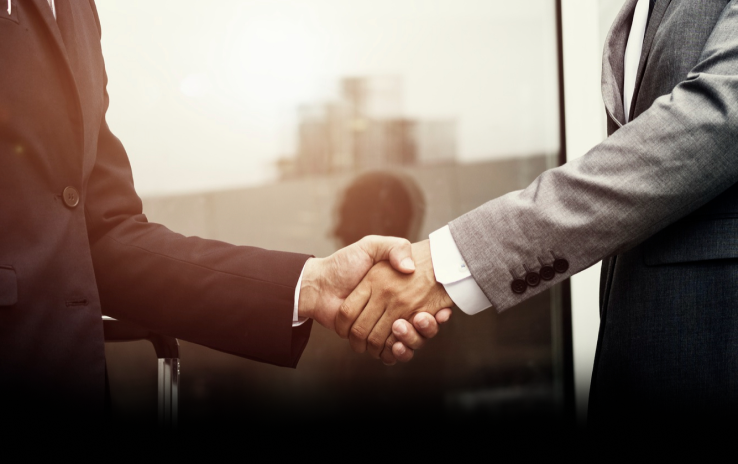